zarnitsa package
Zarnitsa package with data augmentation tools.
- Internal data augmentation using existed data
- External data augmentation setting known statistical distributions by yourself
- NLP augmentation
Principal scheme of project (currently)
Requirements
- Python3
- numpy
- pandas
- nlpaug
- wget
- scikt-learn
Installation
Install package using PyPI:
pip install zarnitsa
Or using actual github repo:
pip install git+https://github.com/AlexKay28/zarnitsa
Usage
Simple usage examples:
Augmentation internal.
This is type of augmentation which you may use in case of working with numerical features.
>>> from zarnitsa.DataAugmenterInternally import DataAugmenterInternally
>>> daug_comb = DataAugmenterInternally()
>>> aug_types = [
>>> "normal",
>>> "uniform",
>>> "permutations",
>>> ]
>>> # pd Series object example
>>> s = pd.Series([1, 2, 3, 4, 5, 6, 7, 8, 9, 10])
>>> for aug_type in aug_types:
>>> print(aug_type)
>>> print(daug_comb.augment_column(s, freq=0.5, return_only_aug=True, aug_type=aug_type))
normal
7 9.958794
3 0.057796
0 -3.135995
6 7.197400
8 13.258087
dtype: float64
uniform
2 10.972232
8 5.335357
9 9.111281
5 5.964971
4 -0.210732
dtype: float64
permutations
4 6
5 4
9 10
3 3
2 5
dtype: int64
Augmentation NLP
This is type of augmentation which you may use in case of working with textual information.
>>> from zarnitsa.DataAugmenterNLP import DataAugmenterNLP
>>> daug = DataAugmenterNLP()
>>> text = "This is sentence example to augment. Thank you for interesting"
>>> daug.augment_column_wordnet(text)
'This be sentence example to augment. Thank you for concern'
>>> daug.augment_column_del(text, reps=1)
'This is sentence example to augment. you for interesting'
>>> daug.augment_column_permut(text, reps=1)
'This sentence is example to augment. Thank you for interesting'
Augmentation External
This is type of augmentation which you may use in case of working with distribution modeling having prior knowlege about it
Doing df[...] = np.nan we imitate data sparsness or misses which we try to fill up using augmentations
>>> size = 500
>>> serial_was = pd.Series(daug.augment_distrib_random(aug_type='normal', loc=0, scale=1, size=size))
>>> serial_new = copy(serial_was)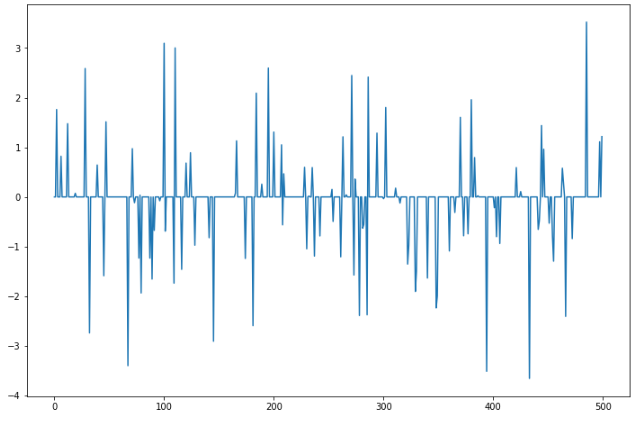
>>> serial_new.loc[serial_new.sample(100).index] = None
>>> serial_new = daug.augment_column(serial_new, aug_type='normal', loc=0, scale=1)
>>> plt.figure(figsize=(12, 8))
>>> serial_was.hist(bins=100)
>>> serial_new.hist(bins=100)
>>> size=50
>>> df = pd.DataFrame({
>>> 'data1': daug.augment_distrib_random(aug_type='normal', loc=0, scale=1, size=size),
>>> 'data2': daug.augment_distrib_random(aug_type='normal', loc=0, scale=1, size=size),
>>> })
>>> for col in df.columns:
>>> df[col].loc[df[col].sample(10).index] = None
>>> plt.figure(figsize=(12, 8))
>>> df.plot()
>>> daug.augment_dataframe(df, aug_type='normal', loc=0, scale=1).plot()